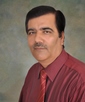
S. N. Jha
ICAR-Central Institute of Post-Harvest Engineering & Technology
India
Title: Spectroscopic method for detection and quantification of food adulterants and contaminants
Biography
Biography: S. N. Jha
Abstract
Food safety is an increasingly important public health issue. It refers to hazards that may make food injurious to the health. The rising incidences of food adulteration and contamination have shaken the trust of consumers. Globalization of food trade, food safety has now become a common concern among both developed and developing countries. Governments round the Globe are intensifying their efforts to improve food safety. Food quality standards have been laid down by many countries to tackle the food safety issues. The food industries therefore need rapid, reliable and affordable technique for food quality analyses to facilitate instant decision making process. Spectroscopy method is an important tool which can easily be employed for high throughput on-line detection of food quality. Infrared (NIR, FTIR) spectroscopy has demonstrated potential as a rapid quality monitoring method for detection of adulterants and contaminants in milk (soymilk, urea, detergent, oil, sugar Aflatoxin B1, M1), fruit juices (patulin, added sugar content, total soluble solids and real juice content and total microbial load on mango surface. FTIR with chemometrics was also used to identify spectral windows having potential of classification of pathogenic bacteria specific to poultry meat. The spectra of food material with and without adulterants and contaminants revealed clear differences based on level and nature of adulterants/ contaminants. Principal component analysis (PCA) showed potential in differentiating the samples at 5% significance level. Pattern recognition analysis using Soft Independent Modeling of Class Analogy (SIMCA) could successfully classify the adulterated/contaminated and non-adulterated/uncontaminated test samples into their respective classes.